신경망(Neural Networks) 과 딥 러닝(Deep Learning) 은 현재 인공 지능(Artificial Intelligence) 과 함께 사용되는 두 가지 핫 화두입니다 . 최근 인공지능 세계의 발전은 이 두 가지가 인공지능의 지능을 향상시키는 데 중요한 역할을 했기 때문이라고 할 수 있습니다.
주위를 둘러보면 점점 더 지능적인 기계를 찾을 수 있을 것입니다. Neural Networks 와 Deep Learning 덕분에 한때 인간의 강점으로 여겨졌던 직무와 능력이 이제는 기계가 수행하고 있습니다. 오늘날 기계는 더 이상 복잡한 알고리즘을 먹도록 만들어지지 않고, 대신 많은 산업 분야에 혁명을 일으킬 수 있는 자율적 독학 시스템으로 발전하기 위해 공급됩니다.
신경망(Neural Networks) 과 딥 러닝(Deep Learning ) 은 이미지 인식, 음성 인식, 데이터 세트에서 더 깊은 관계 찾기와 같은 작업에서 연구자에게 엄청난 성공을 가져다주었습니다. 방대한 양의 데이터와 연산 능력의 도움을 받아 기계는 사물을 인식하고, 음성을 번역하고, 복잡한 패턴을 식별하도록 스스로 훈련하고, 전략을 고안하고 실시간으로 비상 계획을 세우는 방법을 배울 수 있습니다.
이것이 정확히 어떻게 작동합니까? Neutral Networks 와 Deep-Learning 모두 관련이 있다는 사실을 알고 계십니까? 실제로 Deep learning을 이해하려면 먼저 Neural Networks 에 대해 이해해야 합니다 . 자세히 알아보려면 계속 읽으십시오.
신경망이란
신경망(Neural) 은 기본적으로 컴퓨터가 관찰 데이터에서 학습할 수 있도록 하는 프로그래밍 패턴 또는 알고리즘 세트입니다 . 신경망(Neural) 은 패턴을 인식하여 작동 하는 인간의 두뇌와 유사합니다. 감각 데이터는 기계 인식, 레이블 지정 또는 원시 입력 클러스터링을 사용하여 해석됩니다. 인식된 패턴은 이미지, 사운드, 텍스트 등과 같은 데이터가 변환되는 벡터로 묶인 숫자입니다.
Think Neural Network! Think how a human brain function
위에서 언급했듯이 신경망은 인간의 두뇌와 같은 기능을 합니다. 학습 과정을 통해 모든 지식을 습득합니다. 그 후, 시냅스 가중치는 획득한 지식을 저장합니다. 학습 과정에서 네트워크의 시냅스 가중치는 원하는 목표를 달성하기 위해 재구성됩니다.
인간의 두뇌와 마찬가지로 신경망(Neural Networks) 은 패턴 인식 및 인식과 같은 계산을 빠르게 수행하는 비선형 병렬 정보 처리 시스템처럼 작동합니다. 결과적으로 이러한 네트워크는 입력/신호가 본질적으로 비선형인 음성, 오디오 및 이미지 인식과 같은 영역에서 매우 잘 수행됩니다.
간단히 말해서 신경망은 인간의 두뇌처럼 지식을 축적할 수 있는 것으로 기억하고 이를 사용하여 예측할 수 있습니다.(In simple words, you can remember Neural Network as something which is capable of stocking knowledge like a human brain and use it to make predictions.)
신경망의 구조
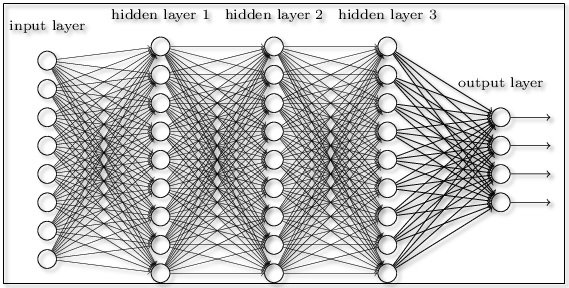
(이미지 크레디트: Mathworks)
신경망 은 3개의 레이어로 구성되며 ,(Networks)
- 입력 레이어,
- 은닉층과
- 출력 레이어.
아래 다이어그램에서 작은 원으로 표시된 것처럼 각 레이어는 하나 이상의 노드로 구성됩니다. 노드 사이의 선은 한 노드에서 다음 노드로의 정보 흐름을 나타냅니다. 정보는 입력에서 출력으로, 즉 왼쪽에서 오른쪽으로 흐릅니다(어떤 경우에는 오른쪽에서 왼쪽 또는 양방향일 수 있음).
입력 레이어의 노드는 수동적이므로 데이터를 수정하지 않습니다. 그들은 입력에서 단일 값을 받고 값을 여러 출력에 복제합니다. 반면(Whereas) , 은닉층과 출력층의 노드는 활성화되어 있습니다. 따라서 그들은 데이터를 수정할 수 있습니다.
상호 연결된 구조에서 입력 레이어의 각 값은 복제되어 모든 은닉 노드로 전송됩니다. 숨겨진 노드에 입력되는 값은 프로그램에 저장된 미리 결정된 숫자 집합인 가중치로 곱해집니다. 그런 다음 가중 입력을 추가하여 단일 숫자를 생성합니다. 신경망은 레이어 수와 레이어당 노드 수에 제한이 없습니다. 대부분의 응용 프로그램은 최대 수백 개의 입력 노드가 있는 3계층 구조를 사용합니다.
신경망의 예(Example of Neural Network)
수중 음파 탐지기 신호에서 물체를 인식하는 신경망을 생각해 보십시오. PC에는 5000개의 신호 샘플이 저장되어 있습니다. PC는 이 샘플이 잠수함, 고래, 빙산, 바다 바위를 나타내는지 아니면 아무 것도 나타내는지 알아내야 합니까? 기존 DSP(Conventional DSP) 방법은 상관 관계 및 주파수 스펙트럼 분석과 같은 수학 및 알고리즘을 사용하여 이 문제에 접근합니다.
신경망을 사용하는 동안 5000개의 샘플이 입력 레이어에 공급되어 출력 레이어에서 값이 튀어나옵니다. 적절한 가중치를 선택하면 광범위한 정보를 보고하도록 출력을 구성할 수 있습니다. 예를 들어, 잠수함(예/아니요), 바다 바위(예/아니요), 고래(예/아니요) 등에 대한 출력이 있을 수 있습니다.
다른 가중치를 사용하여 출력은 물체를 금속 또는 비금속, 생물학적 또는 비생물학적, 적 또는 동맹 등으로 분류할 수 있습니다. 알고리즘, 규칙, 절차가 없습니다. 선택한 가중치 값에 의해 결정되는 입력과 출력 간의 관계만.
이제 딥러닝의 개념을 이해합시다.(Now, let’s understand the concept of Deep Learning.)
딥러닝이란
딥 러닝은 기본적으로 신경망(Neural Networks) 의 하위 집합입니다 . 아마도 많은 숨겨진 레이어가 있는 복잡한 신경망(Neural Network) 이라고 말할 수 있습니다 .
기술적으로 말하면 딥(Deep) 러닝은 신경망 학습을 위한 강력한 기술 세트로 정의할 수도 있습니다. 복잡한 훈련 모델을 가능하게 하기 위해 많은 계층, 방대한 데이터 세트, 강력한 컴퓨터 하드웨어로 구성된 인공 신경망( ANN )을 말합니다. (ANN)여기에는 점점 더 풍부한 기능을 갖춘 여러 계층의 인공 신경망을 사용하는 방법 및 기술 클래스가 포함됩니다.
딥러닝 네트워크의 구조(Structure of Deep learning network)
딥(Deep) 러닝 네트워크는 주로 신경망 아키텍처를 사용하므로 종종 심층 신경망이라고 합니다. "깊은" 작업의 사용은 신경망의 숨겨진 레이어 수를 나타냅니다. 기존 신경망은 3개의 은닉층을 포함하는 반면, 심층 네트워크는 120~150개까지 가질 수 있습니다.
딥 (Deep) 러닝(Learning) 은 컴퓨터 시스템에 다른 데이터에 대한 결정을 내리는 데 사용할 수 있는 많은 데이터를 제공하는 것을 포함합니다. 이 데이터는 머신 러닝의 경우와 마찬가지로 신경망을 통해 제공됩니다. 딥(Deep) 러닝 네트워크는 수동으로 기능을 추출할 필요 없이 데이터에서 직접 기능을 학습할 수 있습니다.
딥 러닝의 예(Examples of Deep Learning)
딥 러닝은 현재 자동차(Automobile) , 항공 우주(Aerospace) 및 자동화(Automation) 에서 의료(Medical) 에 이르기까지 거의 모든 산업에서 활용되고 있습니다. 다음은 몇 가지 예입니다.
- Google , Netflix 및 Amazon : Google 은 음성 및 이미지 인식 알고리즘에서 이를 사용합니다. Netflix 와 Amazon 도 딥 러닝을 사용하여 다음에 시청하거나 구매할 콘텐츠를 결정합니다.
- 운전자 없이 운전: 연구원들은 딥 러닝 네트워크를 활용하여 정지 신호 및 신호등과 같은 물체를 자동으로 감지하고 있습니다. 딥(Deep) 러닝은 보행자를 감지하는 데도 사용되어 사고를 줄이는 데 도움이 됩니다.
- 항공우주 및 방위: 딥 러닝은 관심 영역을 찾는 위성의 물체를 식별하고 군대를 위한 안전하거나 안전하지 않은 영역을 식별하는 데 사용됩니다.
- 딥 러닝(Deep Learning) 덕분에 Facebook 은(Facebook) 자동으로 사진에서 친구를 찾고 태그를 지정합니다. Skype는 음성 커뮤니케이션을 실시간으로 매우 정확하게 번역할 수 있습니다.
- 의학 연구: 의학 연구자들은 딥 러닝을 사용하여 암 세포를 자동으로 감지하고 있습니다.
- 산업 자동화(Industrial Automation) : 딥 러닝은 사람이나 물체가 기계에서 안전하지 않은 거리 내에 있을 때 자동으로 감지하여 중장비 주변의 작업자 안전을 개선하는 데 도움이 됩니다.
- 전자공학: 딥(Deep) 러닝은 자동 청력 및 음성 번역에 사용되고 있습니다.
읽기(Read) : 머신 러닝과 딥 러닝(Machine Learning and Deep Learning) 이란 무엇입니까 ?
결론(Conclusion)
신경망(Neural Networks) 의 개념은 새로운 것이 아니며 연구자들은 지난 10여 년 동안 중간 정도의 성공을 거두었습니다. 그러나 진정한 판도를 바꾼 것은 심층(Deep) 신경망의 진화였습니다.
전통적인 머신 러닝 접근 방식을 능가함으로써 딥 뉴럴 네트워크가 소수의 연구원에 의해 훈련되고 시험될 수 있을 뿐만 아니라 가까운 장래에 더 나은 혁신을 제공하기 위해 다국적 기술 회사에서 채택할 수 있는 범위가 있음을 보여주었습니다.
Thanks to Deep Learning and Neural Network, AI is not just doing the tasks, but it has started to think!
What is Deep Learning and Neural Network
Neural Networks and Deep Learning are currently the two hot buzzwords that are being used nowadays with Artificial Intelligence. The recent developments in the world of Artificial intelligence can be attributed to these two as they have played a significant role in improving the intelligence of AI.
Look around, and you will find more and more intelligent machines around. Thanks to Neural Networks and Deep Learning, jobs and capabilities that were once considered the forte of humans are now being performed by machines. Today, Machines are no longer made to eat more complex algorithms, but instead, they are fed to develop into an autonomous, self-teaching system capable of revolutionizing many industries all around.
Neural Networks and Deep Learning have lent enormous success to the researchers in tasks such as image recognition, speech recognition, finding deeper relations in a data sets. Aided by the availability of massive amounts of data and computational power, machines can recognize objects, translate speech, train themselves to identify complex patterns, learn how to devise strategies and make contingency plans in real-time.
So, how exactly does this work? Do you know that both Neutral Networks and Deep-Learning related, in fact, to understand Deep learning, you must first understand about Neural Networks? Read on to know more.
What is a Neural Network
A Neural network is basically a programming pattern or a set of algorithms that enables a computer to learn from the observational data. A Neural network is similar to a human brain, which works by recognizing the patterns. The sensory data is interpreted using a machine perception, labeling or clustering raw input. The patterns recognized are numerical, enclosed in vectors, into which the data such are images, sound, text, etc. are translated.
Think Neural Network! Think how a human brain function
As mentioned above, a neural network functions just like a human brain; it acquires all the knowledge through a learning process. After that, synaptic weights store the acquired knowledge. During the learning process, the synaptic weights of the network are reformed to achieve the desired objective.
Just like the human brain, Neural Networks work like non-linear parallel information-processing systems which rapidly perform computations such as pattern recognition and perception. As a result, these networks perform very well in areas like speech, audio and image recognition where the inputs/signals are inherently nonlinear.
In simple words, you can remember Neural Network as something which is capable of stocking knowledge like a human brain and use it to make predictions.
Structure of Neural Networks
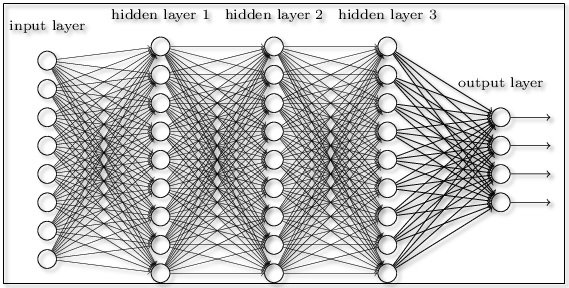
(Image Credit: Mathworks)
Neural Networks comprises of three layers,
- Input layer,
- Hidden layer, and
- Output layer.
Each layer consists of one or more nodes, as shown in the below diagram by small circles. The lines between the nodes indicate the flow of information from one node to the next. The information flows from the input to the output, i.e. from left to right (in some cases it may be from right to left or both ways).
The nodes of the input layer are passive, meaning they do not modify the data. They receive a single value on their input and duplicate the value to their multiple outputs. Whereas, the nodes of the hidden and output layer are active. Thus that can they modify the data.
In an interconnected structure, each value from the input layer is duplicated and sent to all of the hidden nodes. The values entering a hidden node are multiplied by weights, a set of predetermined numbers stored in the program. The weighted inputs are then added to produce a single number. Neural networks can have any number of layers, and any number of nodes per layer. Most applications use the three-layer structure with a maximum of a few hundred input nodes
Example of Neural Network
Consider a neural network recognizing objects in a sonar signal, and there are 5000 signal samples stored in the PC. The PC has to figure out if these samples represent a submarine, whale, iceberg, sea rocks, or nothing at all? Conventional DSP methods would approach this problem with mathematics and algorithms, such as correlation and frequency spectrum analysis.
While with a neural network, the 5000 samples would be fed to the input layer, resulting in values popping from the output layer. By selecting the proper weights, the output can be configured to report a wide range of information. For instance, there might be outputs for: submarine (yes/no), sea rock (yes/no), whale (yes/no), etc.
With other weights, the outputs can classify the objects as metal or non-metal, biological or non-biological, enemy or ally, etc. No algorithms, no rules, no procedures; only a relationship between the input and output dictated by the values of the weights selected.
Now, let’s understand the concept of Deep Learning.
What is a Deep Learning
Deep learning is basically a subset of Neural Networks; perhaps you can say a complex Neural Network with many hidden layers in it.
Technically speaking, Deep learning can also be defined as a powerful set of techniques for learning in neural networks. It refers to artificial neural networks (ANN) that are composed of many layers, massive data sets, powerful computer hardware to make complicated training model possible. It contains the class of methods and techniques that employ artificial neural networks with multiple layers of increasingly richer functionality.
Structure of Deep learning network
Deep learning networks mostly use neural network architectures and hence are often referred to as deep neural networks. Use of work “deep” refers to the number of hidden layers in the neural network. A conventional neural network contains three hidden layers, while deep networks can have as many as 120- 150.
Deep Learning involves feeding a computer system a lot of data, which it can use to make decisions about other data. This data is fed through neural networks, as is the case in machine learning. Deep learning networks can learn features directly from the data without the need for manual feature extraction.
Examples of Deep Learning
Deep learning is currently being utilized in almost every industry starting from Automobile, Aerospace, and Automation to Medical. Here are some of the examples.
- Google, Netflix, and Amazon: Google uses it in its voice and image recognition algorithms. Netflix and Amazon also use deep learning to decide what you want to watch or buy next
- Driving without a driver: Researchers are utilizing deep learning networks to automatically detect objects such as stop signs and traffic lights. Deep learning is also used to detect pedestrians, which helps decrease accidents.
- Aerospace and Defense: Deep learning is used to identify objects from satellites that locate areas of interest, and identify safe or unsafe zones for troops.
- Thanks to Deep Learning, Facebook automatically finds and tags friends in your photos. Skype can translate spoken communications in real-time and pretty accurately too.
- Medical Research: Medical researchers are using deep learning to automatically detect cancer cells
- Industrial Automation: Deep learning is helping to improve worker safety around heavy machinery by automatically detecting when people or objects are within an unsafe distance of machines.
- Electronics: Deep learning is being used in automated hearing and speech translation.
Read: What is Machine Learning and Deep Learning?
Conclusion
The concept of Neural Networks is not new, and researchers have met with moderate success in the last decade or so. But the real game-changer has been the evolution of Deep neural networks.
By out-performing the traditional machine learning approaches it has showcased that deep neural networks can be trained and trialed not just by few researchers, but it has the scope to be adopted by multinational technology companies to come with better innovations in the near future.
Thanks to Deep Learning and Neural Network, AI is not just doing the tasks, but it has started to think!