4차 산업혁명의 기반은 데이터(Data) 와 연결성(Connectivity) 에 크게 좌우될 것 입니다. 데이터 마이닝 솔루션을 개발하거나 생성할 수 있는 Analysis Services(Analysis Services) 는 이와 관련하여 핵심적인 역할을 할 것입니다. 잠재 구매자를 타겟팅하기 위한 고객 구매 행동의 결과를 분석하고 예측하는 데 도움이 될 수 있습니다. 데이터(Data) 는 새로운 천연 자원이 될 것이며 이 정렬되지 않은 데이터에서 관련 정보를 추출하는 프로세스가 매우 중요해질 것입니다. 따라서 데이터 마이닝(Data Mining) 이라는 용어 , 해당 프로세스 및 응용 프로그램에 대한 적절한 이해는 이 유행어에 대한 전체론적 접근 방식을 개발하는 데 도움이 될 수 있습니다.
데이터 마이닝 기초(Data Mining Basics) 및 기술
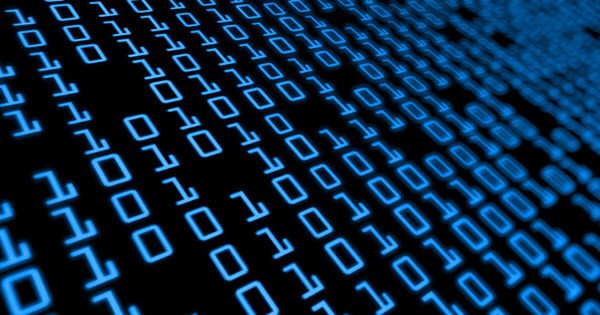
KDD ( Knowledge Discovery in Data ) 라고도 하는 데이터 마이닝 은 대규모 데이터 저장소를 검색하여 단순한 분석을 넘어 패턴과 추세를 파악하는 것입니다. 그러나 이것은 단일 단계 솔루션이 아니라 다단계 프로세스이며 다양한 단계에서 완료됩니다. 여기에는 다음이 포함됩니다.
1] 데이터 수집 및 준비
그것은 데이터 수집과 적절한 조직으로 시작됩니다. 이는 데이터 마이닝을 통해 발견할 수 있는 정보를 찾을 가능성을 크게 높이는 데 도움이 됩니다.
2] 모델 구축 및 평가
데이터 마이닝 프로세스의 두 번째 단계는 다양한 모델링 기법을 적용하는 것입니다. 매개변수를 최적의 값으로 보정하는 데 사용됩니다. 사용되는 기술은 조직의 다양한 요구 사항을 해결하고 결정에 도달하는 데 필요한 분석 기능에 크게 의존합니다.
몇 가지 데이터 마이닝 기술을 간략하게 살펴보겠습니다. 대부분의 조직은 둘 이상의 데이터 마이닝 기술을 결합하여 비즈니스 요구 사항을 충족하는 적절한 프로세스를 형성하는 것으로 나타났습니다.
읽기(Read) : 빅 데이터란 무엇입니까?(What is Big Data?)
데이터 마이닝 기술
- 연관 – (Association – )연관(Association) 은 널리 알려진 데이터 마이닝 기술 중 하나입니다. 이에 따라 동일한 트랜잭션 내 항목 간의 관계를 기반으로 패턴을 해독합니다. 따라서(Hence) 관계 기술이라고도 합니다. 대형 브랜드 소매업체는 이 기술을 사용하여 고객의 구매 습관/선호도를 조사합니다. 예를 들어, 사람들의 구매 습관을 추적할 때 소매업체는 고객이 초콜릿을 구입할 때 항상 크림을 구매한다는 것을 식별하고 다음번에 초콜릿을 구입할 때 크림도 사고 싶어할 수 있다고 제안할 수 있습니다.
- 분류 – 이 데이터 마이닝 기법은 기계 학습을 기반으로 하고 (Classification)선형(Linear) 프로그래밍, 의사 결정(Decision) 트리, 신경망(Neural) 과 같은 수학적 기법을 사용한다는 점에서 위와 다릅니다.회로망. 분류에서 기업은 데이터 항목을 그룹으로 분류하는 방법을 배울 수 있는 소프트웨어를 구축하려고 합니다. 예를 들어, 회사는 "퇴사를 제안한 직원의 모든 기록을 고려하여 미래에 회사를 사임할 가능성이 있는 개인의 수를 예측"하는 애플리케이션의 분류를 정의할 수 있습니다. 이러한 시나리오에서 회사는 직원의 기록을 "퇴사"와 "체류"의 두 그룹으로 분류할 수 있습니다. 그런 다음 데이터 마이닝 소프트웨어를 사용하여 직원을 이전에 만든 별도의 그룹으로 분류할 수 있습니다.
- 클러스터링(Clustering) – 다름(Different)유사한 특성을 나타내는 개체를 자동화를 통해 단일 클러스터로 그룹화합니다. 그러한 많은 클러스터는 클래스와 객체(유사한 특성을 가진)가 그에 따라 배치될 때 생성됩니다. 이를 더 잘 이해하기 위해 도서관에서 책을 관리하는 예를 살펴보겠습니다. 도서관에는 방대한 도서 컬렉션이 완전히 목록화되어 있습니다. 같은 유형의 항목이 함께 나열됩니다. 이렇게 하면 관심 있는 책을 더 쉽게 찾을 수 있습니다. 유사하게, 클러스터링 기술을 사용하여 일종의 유사성을 가진 책을 하나의 클러스터에 보관하고 적절한 이름을 지정할 수 있습니다. 따라서 독자가 자신의 관심과 관련된 책을 찾으려면 전체 라이브러리를 검색하는 대신 해당 책장으로 이동하기만 하면 됩니다. 따라서 클러스터링 기술은 클래스를 정의하고 각 클래스에 객체를 넣습니다.
- 예측(Prediction) – 예측은 다른 데이터 마이닝 기술과 함께 자주 사용되는 데이터 마이닝 기술입니다. 추세, 분류, 패턴 일치 및 관계 분석이 포함됩니다. 과거 사건이나 사건을 적절한 순서로 분석함으로써 미래 사건을 안전하게 예측할 수 있습니다. 예를 들어, 판매를 독립 변수로 선택하고 이익을 판매 종속 변수로 선택하면 예측 분석 기법을 판매에 사용하여 미래 이익을 예측할 수 있습니다. 그런 다음 과거 판매 및 이익 데이터를 기반으로 이익 예측에 사용되는 적합 회귀 곡선을 그릴 수 있습니다.
- 의사 결정 트리(Decision trees) – 의사 결정 트리 내에서 여러 답변이 있는 간단한 질문으로 시작합니다. 각 답변은 데이터를 분류하거나 식별하는 데 도움이 되는 추가 질문으로 연결되어 데이터가 분류될 수 있도록 하거나 각 답변을 기반으로 예측할 수 있도록 합니다. 예를 들어, 우리는 다음 결정 트리를 사용하여 크리켓 경기를 할지 여부를 결정합니다 . ODI : 데이터 마이닝 결정 트리(Data Mining Decision Tree) : 루트 노드에서 시작하여 일기예보가 비를 예측하면 당일 경기를 피해야 합니다. 또는 일기예보가 맑으면 경기를 해야 합니다.
데이터 마이닝 은 커뮤니케이션, (Data Mining)보험(Insurance) , 교육(Education) , 제조(Manufacturing) , 은행(Banking) , 소매(Retail) 등 다양한 산업과 분야에 걸친 분석 노력의 핵심입니다 . 따라서 다른 기술을 적용하기 전에 이에 대한 올바른 정보를 갖는 것이 중요합니다.
What is Data Mining? Basics and its Techniques.
The fоundation of the fourth industrial revolution will largely depend upon Data and Connectivity. Analysis Services capable of developing or creating data mining solutions will play a key role in this regard. It could assist in analyzing and predicting outcomes of customer purchasing behavior for targeting potential buyers. Data will become a new natural resource and the process of extracting relevant information from this unsorted data will assume immense importance. As such, a proper understanding of the term – Data Mining, its processes, and application could help us in developing a holistic approach to this buzzword.
Data Mining Basics and its Techniques
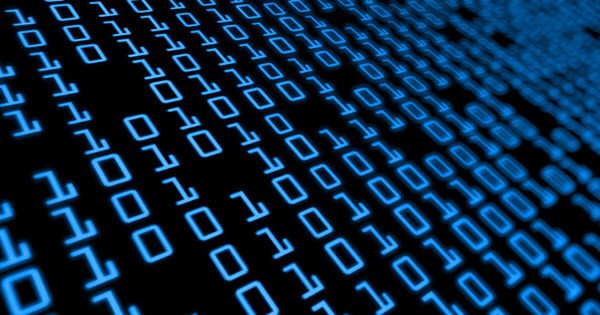
Data mining, also known as Knowledge Discovery in Data (KDD) is about searching large stores of data to uncover patterns and trends that go beyond simple analysis. This, however, is not a single-step solution but a multi-step process and is completed in various stages. These include:
1] Data gathering and Preparation
It starts with data collection and its proper organization. This helps in significantly improving the chances of finding the information that can be discovered through data mining
2] Model Building and Evaluation
The second step in data mining process is the application of various modeling techniques. These are used to calibrate the parameters to optimal values. Techniques employed largely depend on analytic capabilities required to address a gamut of organizational needs and to arrive at a decision.
Let us examine some data mining techniques in brief. It is found that most organizations combine two or more data mining techniques together to form an appropriate process that meets their business requirements.
Read: What is Big Data?
Data Mining Techniques
- Association – Association is one of the widely-known data mining techniques. Under this, a pattern is deciphered based on a relationship between items in the same transaction. Hence, it is also known as the relation technique. Big brand retailers rely on this technique to research customer’s buying habits/preferences. For example, when tracking people’s buying habits, retailers might identify that a customer always buys cream when they buy chocolates, and therefore suggest that the next time that they buy chocolates they might also want to buy cream.
- Classification – This data mining technique differs from the above in the way that it is based on machine learning and uses mathematical techniques such as Linear programming, Decision trees, Neural network. In classification, companies try to build software that can learn how to classify the data items into groups. For instance, a company can define a classification in the application that “given all records of employees who offered to resign from the company, predict the number of individuals who are likely to resign from the company in future.” Under such a scenario, the company can classify the records of employees into two groups that namely “leave” and “stay”. It can then use its data mining software to classify the employees into separate groups created earlier.
- Clustering – Different objects exhibiting similar characteristics are grouped together in a single cluster via automation. Many such clusters are created as classes and objects (with similar characteristics) are placed in it accordingly. To understand this better, let us consider an example of book management in the library. In a library, the vast collection of books is fully cataloged. Items of the same type are listed together. This makes it easier for us to find a book of our interest. Similarly, by using the clustering technique, we can keep books that have some kinds of similarities in one cluster and assign it a suitable name. So, if a reader is looking to grab a book relevant to his interest, he only has to go to that shelf instead of searching the entire library. Thus, the clustering technique defines the classes and puts objects in each class, while in the classification techniques, objects are assigned into predefined classes.
- Prediction – The prediction is a data mining technique that is often used in combination with the other data mining techniques. It involves analyzing trends, classification, pattern matching, and relation. By analyzing past events or instances in a proper sequence one can safely predict a future event. For instance, the prediction analysis technique can be used in the sale to predict future profit if the sale is chosen as an independent variable and profit as a variable dependent on sale. Then, based on the historical sale and profit data, one can draw a fitted regression curve that is used for profit prediction.
- Decision trees – Within the decision tree, we start with a simple question that has multiple answers. Each answer leads to a further question to help classify or identify the data so that it can be categorized, or so that a prediction can be made based on each answer. For example, We use the following decision tree to determine whether or not to play cricket ODI: Data Mining Decision Tree: Starting at the root node, if the weather forecast predicts rain then, we should avoid the match for the day. Alternatively, if the weather forecast is clear, we should play the match.
Data Mining is at the heart of analytics efforts across a variety of industries and disciplines like communications, Insurance, Education, Manufacturing, Banking and Retail and more. Therefore, having correct information about it is essential before apply the different techniques.